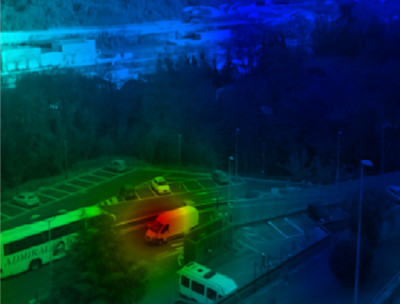
Dataset for object tracking using both sound and video data. The proposed dataset is composed by 3 different sequences of audio-video data, collected with the DualCam device in both indoor and outdoor scenarios: (1) Drone Sequence; (2) Voice Sequence; and (3) Motorbike Sequence.
The aim is to show the potentialities of using acoustic images for target tracking in three challenging scenarios. In particular, the audio-based approach, proposed in the paper, is able to overcome, often dramatically, visual tracking with state-of-art algorithms, dealing efficiently with occlusions, abrupt variations in visual appearence and camouflage. These results pave the way to a widespread use of acoustic imaging in application scenarios such as in security and surveillance.
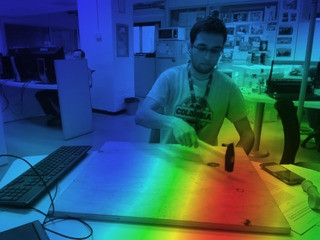
A new multimodal dataset comprised of visual data as RGB images and acoustic data as raw audio signals acquired from 128 microphones and multispectral acoustic images generated by a filter-and-sum beamforming algorithm. The dataset consists of 378 audio-visual sequences between 30 and 60 seconds depicting different people performing individually a set of actions that produce a characteristic sound. The provided visual and acoustic images are both aligned in space and synchronized in time.
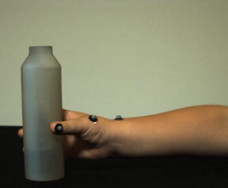
We propose here a new video dataset consisting in a set of video clips of reach-to-grasp actions performed by children with Autism Spectrum Disorders (ASD) and IQ-matched typically developing (TD) children. Children of the two groups were asked to grasp a bottle, in order to perform four different subsequent actions (placing, pouring, passing to pour, and passing to place). Motivated by recent studies in psychology and neuroscience, we attempt to classify whether actions are performed by a TD or an ASD child, by only processing the part of video data recording the grasping gesture. In our work the only exploitable information is conveyed by the kinematics, being the surrounding context totally uninformative. For a detailed description of the problem and the dataset, please refer to the paper.
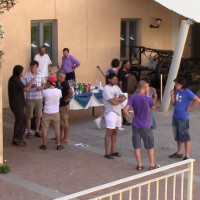
The dataset serves for checking the ability in detecting social interactions, i.e., people that talk with themselves.
The dataset represents a coffee-break scenario of a social event that lasted 4 days, captured by two cameras. The dataset is part of a social signaling project whose aim is to monitor how social relations evolve over time. Nowadays, only 2 sequences of a single day of a single camera have been annotated (but novel sequences are going to appear, keep in touch!). A psychologist annotated the videos indicating the groups present in the scenes, for a total of 45 frames for Seq1 and 75 frames for Seq2. The annotations have been done by analyzing each frame and a set of questionnaires that the subjects filled in. The dataset is still challenging from the tracking and head pose estimation point of view, due to multiple occlusions. Results of this dataset have been published in the referenced papaer.
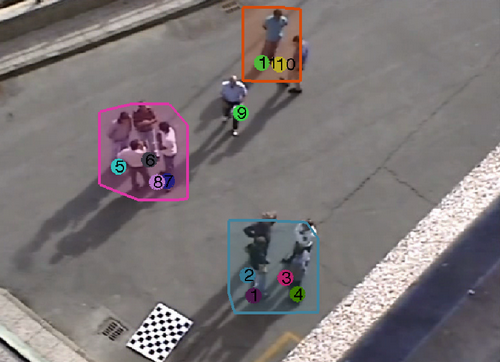
We present a new annotated dataset that contains groups of people that evolve, appear and disappear spontaneously, and experience split and merge events. This correspond to cocktail party-like situations in social areas where people arrive alone or with other people, move from one group to another, stay still while conversing, etc. Such a picture was missing in literature, since most of the existing datasets with labelled groups contain wlaking pedestrian with a main flow direction. We manually annotated people and groups on each frame and over time, i.e., detection and tracking of groups and individuals.
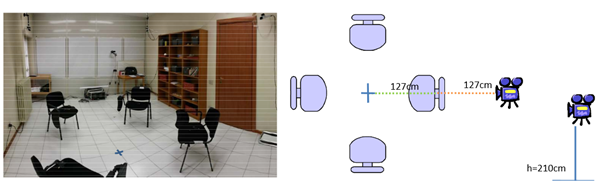
The aim of this corpus is automatically detecting emergent leaders and their leadership styles (autocratic or democratic) in a meeting environment. It contains 16 meeting sessions. The meeting sessions are composed of the same gender, unacquainted four-person (in total 44 females and 20 males) with the average age of 21.6 with 2.24 standard deviation.
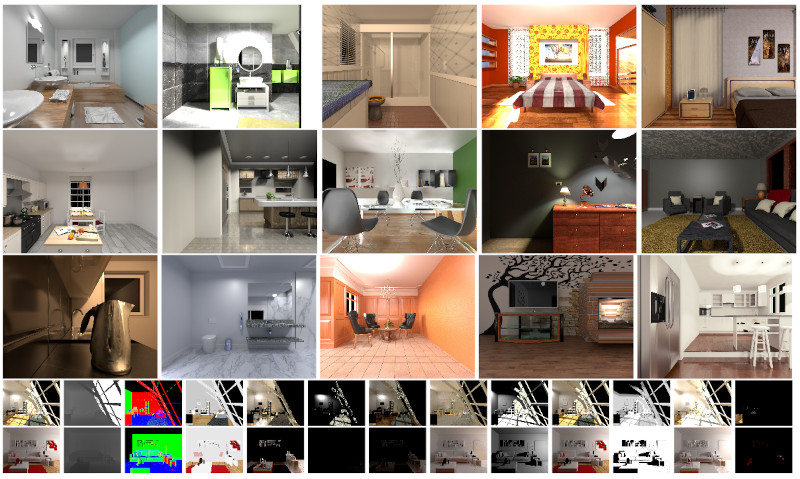
Specular highlights are commonplace in images, however, methods for detecting them and in turn removing the phenomenon are particularly challenging. A reason for this, is due to the difficulty of creating a dataset for training or evaluation, as in the real-world we lack the necessary control over the environment. Therefore, we propose a novel physically-based rendered LIGHT Specularity (LIGHTS) Dataset for the evaluation of the specular highlight detection task. Our dataset consists of 18 high quality architectural scenes, where each scene is rendered with multiple views. In total we have 2,603 views with an average of 145 views per scene.
Mice behavioural analysis plays a key role in neuroscience. In fact, the visual analysis of genetically modified mice allows to get insights about particular genes, phenotypes or drug effects. As part of our research, we have collected hours of mice interactions which can be used for training, testing and validation of many aspects of mouse tracking and general social behaviour classification. The recordings comprise of uncompressed frames captured by a overhead infrared camera. Different numbers of interacting mice (from 2 to 4) and mouse strains are employed.
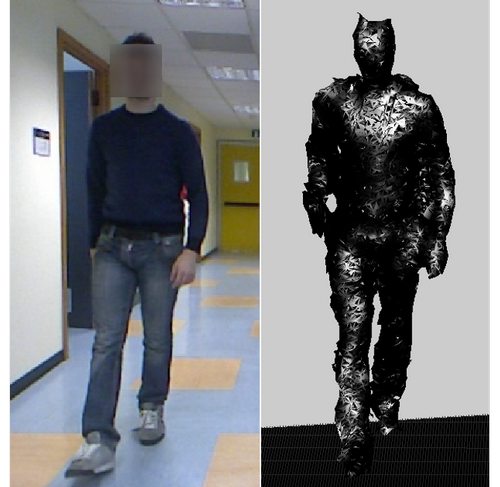
We present a new dataset for person re-identification using depth information. The main motivation is that the standard techniques (such as SDALF) fail when the individuals change their clothing, therefore they cannot be used for long-term video surveillance. Depth information is the solution to deal with this problem because it stays constant for a longer period of time. While several datasets for appearance-based re-identification exist, the literature still misses a dataset that provides also depth. This dataset aims at promoting the RGB-D re-identification research.
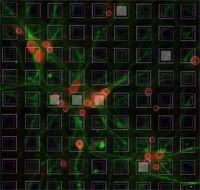
We present 6 different image datasets acquired by fluorescence microscopy and depicting hippocampal in-vitro neuronal networks. The datasets are used for the evaluation of our approach for the joint analysis of neuronal anatomy and functionality, involving the use of a high-resolution Multi-Electrode Array (MEA) technology for the acquisition of the functional signal. Results concerning both the neuronal nuclei detection and the structural/functional representation of the neuron/electrode mapping are provided.
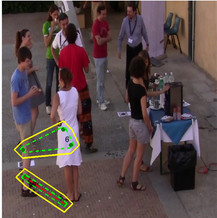
A standing conversational group (also known as F-formation) occurs when two or more people sustain a social interaction, such as chatting at a cocktail party. Detecting such interactions in images or videos is of fundamental importance in many contexts, like surveillance, social signal processing, social robotics or activity classification. This paper presents an approach to this problem which models the socio-psychological concept of an F-formation. Essentially, an F-formation defines some constraints on how subjects have to be mutually located and oriented. We develop a game-theoretic framework, embedding these constraints, which is supported by a statistical modeling of the uncertainty associated with the position and orientation of people.
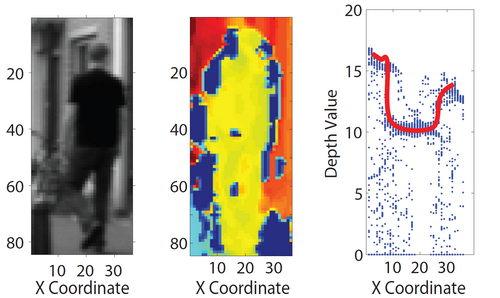
Capturing the essential characteristics of visual objects by considering how their features are inter-related is a recent philosophy of object classification. We embed this principle in a novel image descriptor, dubbed Heterogeneous Auto-Similarities of Characteristics (HASC). HASC is applied to heterogeneous dense features maps, encoding linear relations by covariances and nonlinear associations through information-theoretic measures such as mutual information and entropy. In this way, highly complex structural information can be expressed in a compact, scale invariant and robust manner.
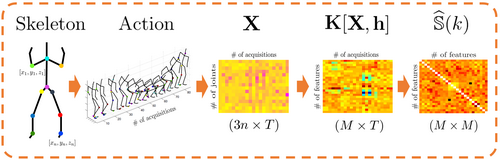
The descriptive power of the covariance matrix is limited in capturing linear mutual dependencies between variables only. To solve this issue, we present a rigorous and principled mathematical pipeline to recover the kernel trick for computing the covariance matrix, enhancing it to model more complex, non-linear relationships conveyed by the raw data. Our proposed encoding (Kernelized-COV) generalizes the original covariance representation without compromising the efficiency of the computation. Despite its broad generability, the aforementioned paper applied Kernelized-COV to 3D action recognition from MoCap data.